![]() |
JOURNAL TOOLS |
Publishing options |
eTOC |
To subscribe |
Submit an article |
Recommend to your librarian |
ARTICLE TOOLS |
Publication history |
Reprints |
Permissions |
Cite this article as |
Share |
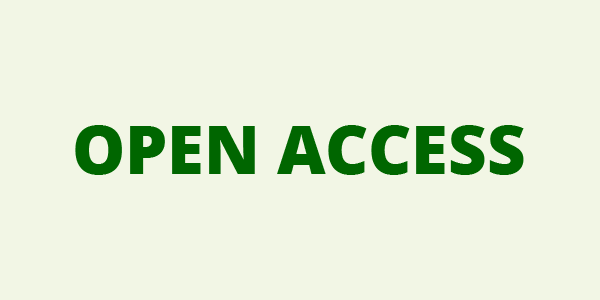
YOUR ACCOUNT
YOUR ORDERS
SHOPPING BASKET
Items: 0
Total amount: € 0,00
HOW TO ORDER
YOUR SUBSCRIPTIONS
YOUR ARTICLES
YOUR EBOOKS
COUPON
ACCESSIBILITY
REVIEW NUCLEAR MEDICINE IN PRECISION ONCOLOGY
The Quarterly Journal of Nuclear Medicine and Molecular Imaging 2020 September;64(3):278-90
DOI: 10.23736/S1824-4785.20.03263-X
Copyright © 2020 EDIZIONI MINERVA MEDICA
language: English
Nuclear medicine radiomics in precision medicine: why we can’t do without artificial intelligence
Wyanne A. NOORTMAN 1, 2 ✉, Dennis VRIENS 1, Willem GROOTJANS 3, Qian TAO 4, Lioe-Fee de GEUS-OEI 1, 2, Floris H. VAN VELDEN 1
1 Section of Nuclear Medicine, Department of Radiology, Leiden University Medical Center (LUMC), Leiden, the Netherlands; 2 Biomedical Photonic Imaging Group, University of Twente, Enschede, the Netherlands; 3 Department of Radiology, Leiden University Medical Center (LUMC), Leiden, the Netherlands; 4 Division of Image Processing (LKEB), Department of Radiology, Leiden University Medical Center (LUMC), Leiden, the Netherlands
In recent years, radiomics, defined as the extraction of large amounts of quantitative features from medical images, has gained emerging interest. Radiomics consists of the extraction of handcrafted features combined with sophisticated statistical methods or machine learning algorithms for modelling, or deep learning algorithms that both learn features from raw data and perform modelling. These features have the potential to serve as non-invasive biomarkers for tumor characterization, prognostic stratification and response prediction, thereby contributing to precision medicine. However, especially in nuclear medicine, variable results are obtained when using radiomics for these purposes. Individual studies show promising results, but due to small numbers of patients per study and little standardization, it is difficult to compare and validate results on other datasets. This review describes the radiomic pipeline, its applications and the increasing role of artificial intelligence within the field. Furthermore, the challenges that need to be overcome to achieve clinical translation are discussed, so that, eventually, radiomics, combined with clinical data and other biomarkers, can contribute to precision medicine, by providing the right treatment to the right patient, with the right dose, at the right time.
KEY WORDS: Nuclear medicine; Diagnostic imaging; Radiography; Artificial intelligence