![]() |
JOURNAL TOOLS |
Publishing options |
eTOC |
To subscribe |
Submit an article |
Recommend to your librarian |
ARTICLE TOOLS |
Publication history |
Reprints |
Permissions |
Cite this article as |
Share |
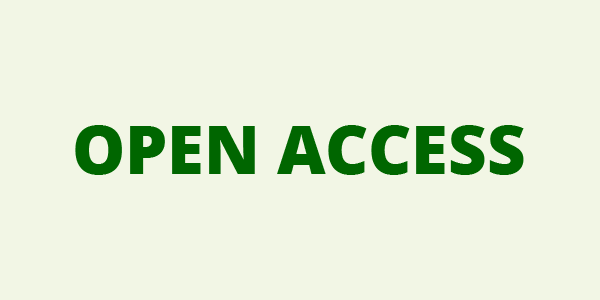
YOUR ACCOUNT
YOUR ORDERS
SHOPPING BASKET
Items: 0
Total amount: € 0,00
HOW TO ORDER
YOUR SUBSCRIPTIONS
YOUR ARTICLES
YOUR EBOOKS
COUPON
ACCESSIBILITY
ORIGINAL ARTICLE NEW PERSPECTIVES IN SCOLIOSIS AND SPINAL DEFORMITIES: AN UPDATE FROM THE 2023 ANNUAL SOSORT MEETING Open access
European Journal of Physical and Rehabilitation Medicine 2023 August;59(4):535-42
DOI: 10.23736/S1973-9087.23.08091-7
Copyright © 2023 THE AUTHORS
This is an open access article distributed under the terms of the CC BY-NC-ND 4.0 license which allows users to copy and distribute the manuscript, as long as this is not done for commercial purposes and further does not permit distribution of the manuscript if it is changed or edited in any way, and as long as the user gives appropriate credits to the original author(s) and the source (with a link to the formal publication through the relevant DOI) and provides a link to the license.
language: English
Validation of an artificial intelligence-based method to automate Cobb angle measurement on spinal radiographs of children with adolescent idiopathic scoliosis
Jason C. WONG 1, Marek Z. REFORMAT 1, Eric C. PARENT 2, Kyle P. STAMPE 3, Sarah C. SOUTHON HRYNIUK 3, Edmond H. LOU 1 ✉
1 Department of Electrical and Computer Engineering, University of Alberta, Edmonton, Canada; 2 Department of Physical Therapy, University of Alberta, Edmonton, Canada; 3 Department of Surgery, University of Alberta, Edmonton, Canada
BACKGROUND: Accurately measuring the Cobb angle on radiographs is crucial for diagnosis and treatment decisions for adolescent idiopathic scoliosis (AIS). However, manual Cobb angle measurement is time-consuming and subject to measurement variation, especially for inexperienced clinicians.
AIM: This study aimed to validate a novel artificial-intelligence-based (AI) algorithm that automatically measures the Cobb angle on radiographs.
DESIGN: This is a retrospective cross-sectional study.
SETTING: The population of patients attended the Stollery Children’s Hospital in Alberta, Canada.
POPULATION: Children who: 1) were diagnosed with AIS, 2) were aged between 10 and 18 years old, 3) had no prior surgery, and 4) had a radiograph out of brace, were enrolled.
METHODS: A total of 330 spinal radiographs were used. Among those, 130 were used for AI model development and 200 were used for measurement validation. Automatic Cobb angle measurements were validated by comparing them with manual ones measured by a rater with 20+ years of experience. Analysis was performed using the standard error of measurement (SEM), inter-method intraclass correlation coefficient (ICC
RESULTS: The AI method detected 346 of 352 manually measured curves (mean±standard deviation: 24.7±9.5°), achieving 91% (316/346) of measurements within clinical acceptance. Excellent reliability was obtained with 0.92 ICC and 0.79° SEM. Comparable performance was found throughout all subgroups, and no systematic biases in performance affecting any subgroup were discovered. The algorithm measured each radiograph approximately 18s on average which is slightly faster than the estimated measurement time of an experienced rater. Radiographs taken by the EOS X-ray system were measured more quickly on average than those taken by a conventional digital X-ray system (10s vs. 26s).
CONCLUSIONS: An AI-based algorithm was developed to measure the Cobb angle automatically on radiographs and yielded reliable measurements quickly. The algorithm provides detailed images on how the angles were measured, providing interpretability that can give clinicians confidence in the measurements.
CLINICAL REHABILITATION IMPACT: Employing the algorithm in practice could streamline clinical workflow and optimize measurement accuracy and speed in order to inform AIS treatment decisions.
KEY WORDS: Adolescent; Scoliosis; Artificial intelligence; Radiography